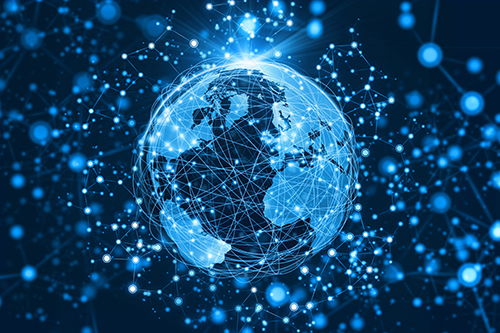
A team of scientists led by researchers at the National Institutes of Health (NIH) reviewed the growing body of research on how big data can impact infectious diseases and has published its analyses in a special issue of The Journal of Infectious Diseases. Big data derived from electronic health records, social media, the internet, and other digital sources has the potential to provide more timely and detailed information on infectious disease threats or outbreaks than traditional surveillance methods.
Traditional infectious disease surveillance typically relies on laboratory tests and other data collected by public health institutions. However, the authors note it can have time lags, is expensive to produce, and typically lacks the local resolution needed for accurate monitoring. Moreover, it can be cost-prohibitive in low-income countries. In contrast, big data streams from internet queries, for example, are available in real time and can track disease activity locally, but have their own biases. Hybrid tools that combine traditional surveillance and big data sets may provide a way forward the scientists suggest, serving to complement, rather than replace, existing methods.
“The ultimate goal is to be able to forecast the size, peak or trajectory of an outbreak weeks or months in advance in order to better respond to infectious disease threats. Integrating big data in surveillance is the first step toward this long-term goal,” explained Cecile Viboud, Ph.D., co-editor of the supplement and a senior scientist at the NIH's Fogarty International Center. “Now that we have demonstrated proof of concept by comparing data sets in high-income countries, we can examine these models in low-resource settings where traditional surveillance is sparse.”
A team of experts in epidemiology, computer science, and modeling collaborated on the supplement's ten articles. They report on the opportunities and challenges associated with three types of data: medical encounter files, such as records from healthcare facilities and insurance claim forms; crowdsourced data collected from volunteers who self-report symptoms in near real time; and data generated through the use of social media, the internet and mobile phones—which may include self-reporting of health, behavior and travel information—to help elucidate disease transmission.
While much of the issue is devoted to the beneficial impact big data could have on disease surveillance, the authors note that big data's potential must be tempered with caution. Non-traditional data streams may lack key demographic identifiers such as age and sex, or provide information that underrepresents infants, children, the elderly. It may also lack data from developing countries. Social media outlets may not be stable sources of data, as they can disappear if there is a loss of interest or financing. Most importantly, any novel data stream must be validated against established infectious disease surveillance data and systems.
While the new hybrid models that combine traditional and digital disease surveillance methods show promise, the scientists agree there is still an overall scarcity of reliable monitoring information, especially compared to other fields such as climatology, where the data sets are huge.
“To be able to produce accurate forecasts, we need better observational data that we just don't have in infectious diseases,” noted Shweta Bansal, Ph.D., assistant professor in the department of biology at Georgetown University, a co-editor of the supplement. “There's a magnitude of difference between what we need and what we have, so our hope is that big data will help us fill this gap.”