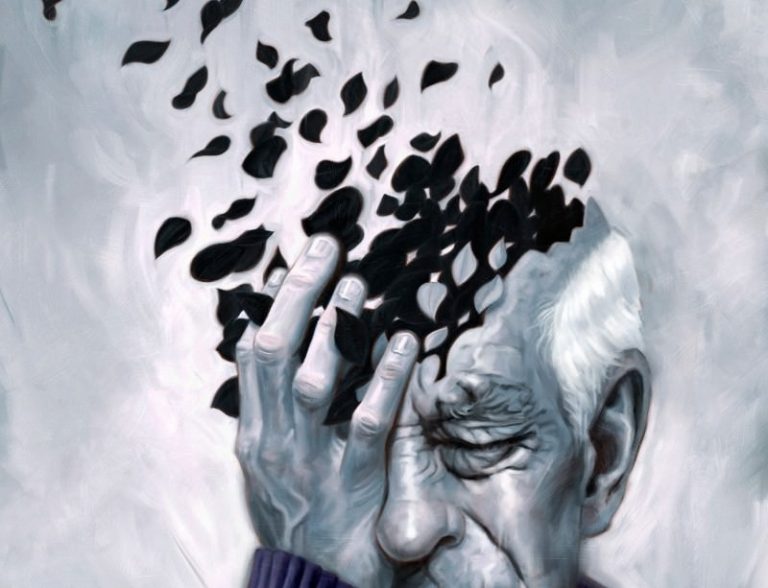
A computational model that predicts brain age using a large collection of FDG-PET (fluorodeoxyglucose positron emission tomography) and structural MRI (magnetic resonance imaging) imaging data has been developed by Mayo Clinic scientists.
The deep learning-based model tests the relationship between brain age gaps in various forms of dementia, including mild cognitive impairment (MCI), Alzheimer’s disease (AD), frontotemporal dementia (FTD), and Lewy body dementia (LBD), as well as in normal brains.
Senior author of the new study, published in the journal Nature Aging, David Jones, an assistant professor at the Mayo Clinic said: “We are building artificial intelligence (AI) capabilities in the department of neurology at Mayo Clinic. We have also been focused on normal aging in the context of Alzheimer’s disease and related disorders for decades. This project is part of these larger efforts to improve the care and understanding of these conditions using artificial intelligence models.”
Earlier studies have established that structural and functional changes occur in the brain as it ages and that these changes accelerate in neurodegenerative diseases.
“Here we investigate the biomarker potential of deep learning-based brain age predictions as it relates to other biomarkers of Alzheimer’s disease and its ability to predict future cognitive decline. These types of investigations are also very informative in terms of the underlying neurobiology of aging and its relationship to degenerative diseases of brain function,” said Jones.
To interpret the models, the researchers performed occlusion analysis. Occlusion denotes structures blocked by others on an image and affects how a deep learning model segments a structure in an image.
“The occlusion analysis attempts to determine how important a region of the brain image is for making an accurate prediction by comparing the prediction with that portion of the imaging included versus the same prediction when the model is not provided the information from that region of the brain,” explained Jones.
“In simple terms, we see how badly the model breaks when we hide information from a portion of the brain. The more the model breaks when we hide information from a particular brain region, the more important that information is for the model’s performance,” he added.
To train the AI model system to predict brain age, the investigators first trained the system on healthy aging trajectories through neuroimaging data from 30 to 97-year-old normal participants. Using occlusion analysis, the team then showed that training the models on the imaging data led the models to learn patterns of brain aging based on the age of the individual and the specificity of the modality.
The team also studied age- and modality-specific saliency maps using occlusion sensitivity analysis for each age group, where higher saliency denotes the region’s importance in estimating brain age.
Jones explained, “Using interpretability experiments we demonstrate that the patterns of brain aging appear anatomically different for images of glucose metabolism as compared to structural imaging with MRI. This advances understanding of the biology of aging and Alzheimer’s disease from the unique perspectives of brain structure and function.”
The investigators found that the increase in the gap of brain age correlated highly with cognitive impairment and the AD biomarkers. A higher gap of brain age also revealed a longitudinal predictive characteristic in all types of dementia examined, although, interestingly, the regions of the brain that generated the differences in brain age, were different for each group of dementia patients.
“The ability for deep learning to accurately predict age based on brain imaging data has been known for some time. However, looking at brain age gap or the difference between predicted and actual age, has been thought to have the potential to be utilized as a biomarker. Others have argued that such a brain age gap is only able to mark treatment-level biological differences and is unable to track changes in state and therefore should not be interpreted as accelerated brain aging,” Jones said. “The main finding of our study is that we could indeed find evidence that high brain age gap is behaving as an accelerated brain aging biomarker.”
Using readouts of brain metabolism from FDG PET scans as inputs for the deep learning model to predict the brain age is a novel approach adopted in this study. In addition, the demonstration that brain metabolic markers are related to established AD biomarkers and predictive of cognitive decline is a novel finding.
In follow-up projects, Jones and his team intend to conduct validation and testing of these methods across diverse clinical and imaging samples in the clinical workflow so that brain age prediction through this AI system can be integrated into the suite of tools used to evaluate patients at Mayo Clinic.