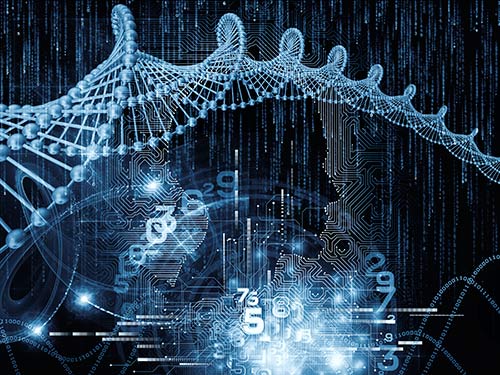
Japanese researchers have applied artificial intelligence to the evolutionary history of an enzyme in order to engineer its biological properties. The machine learning system streamlines the process of by which an enzyme’s constituent amino acids can be successfully reconfigured.
This affects its mode of action on a specific substrate and the “helper” cofactor compounds it needs for its catalytic functions.
The research, published in the journal ACS Synthetic Biology, was successfully tested in the malic enzyme and could provide new insights into substrate-enzyme specificity.
Its potential applications are diverse and could include protein and metabolic engineering, pharmaceuticals and even biofuel production.
“By using artificial intelligence, we identified unexpected amino acid residues in malic enzyme that correspond to the enzyme’s use of different redox cofactors,” explained co-senior author Hiroshi Shimizu, a professor running his own lab studying metabolic engineering integrated with bioinformatics data.
“This helped us understand the substrate specificity mechanism of the enzyme and will facilitate optimal engineering of the enzyme in laboratories.”
Artificially designing an enzyme by altering its specificity for a specific substrate or cofactor can involve multiple mutations at positions around its substrate-binding pocket.
This is difficult enough to unpack, and traditional methods using the enzyme’s crystalline structure can make the process yet more complex and laborious.
The researchers therefore developed a methodology in which amino acids could be ranked based on their sequence in analogous enzymes from other living species.
Specifically, the machine learning system used structurally homologous but functionally distinct amino acid sequences in different species to navigate toward the target function.
The researchers found that the redox cofactor preference of malic enzymes could be converted by applying phylogenetic analysis to machine learning, without experimental screening.
“Since multiple mutagenesis is necessary to alter substrate and cofactor specificity, we believe that [machine learning] can be an effective tool in identifying mutation positions and suggesting candidate amino acids,” they maintain.
The team adds: “While directed evolution is generally limited to a few mutations in a protein, our model allows the introduction of dozens of mutations as if guiding a path through a vast search space of the protein.’