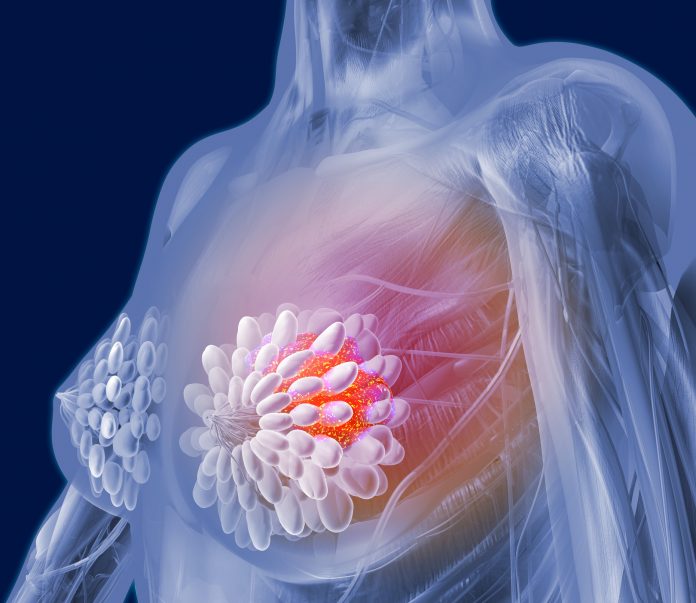
Researchers at the University of Copenhagen have shown that using a commercially available AI system can reduce the workload of radiologists by over 33% while improving the overall performance of breast cancer screenings.
Mammography exams are routinely used for the early detection of breast cancer, which helps reduce the mortality of the disease. However, these exams place “a substantial workload on radiologists, who must read a large number of mammograms, the majority of which don’t warrant a recall of the patient,” said Andreas D. Lauritzen, PhD, a researcher at the University of Copenhagen and Gentofte Hospital in Denmark.
“The reading workload is further compounded when screening programs employ double reading to improve cancer detection and decrease false-positive recalls,” added Lauritzen. Recalling women for further tests also adds to the radiologists’ workload, which can be a challenge given the general shortage of specialized breast radiologists.
In a study published in Radiology, Lauritzen and colleagues investigated the effects that using an AI system to assist with breast cancer screening affected the workload and performance of radiologists.
The researchers trained an AI system called Transpara using deep learning models to identify suspicious lesions within mammograms and give them a score out of 100 indicating how likely it is for the lesion to be cancerous.
The researchers looked at mammograms from women between the ages of 50 and 69 who undertook a routine screening between October 2020 and October 2022 in the Capital Region of Denmark.
In total 60,751 women were screened without using AI, and 58,246 women were screened using the AI system. In the second group, the mammograms were first analyzed by AI. The exams that the AI deemed to have a low likelihood of cancer (67% of screenings) were then read by a specialized breast radiologist to confirm the results. The rest of the exams (33% of screenings) were read by two radiologists with AI-assisted decision support.
In those cases where a cancerous lesion was identified by radiologists, the patients were recalled and the cancers were confirmed with a biopsy. All women in the study were followed for at least 180 days.
The results showed that screening patients with the help of the AI system detected breast cancers in 0.82% of screenings, significantly more than without AI, which detected breast cancer in 0.70% of screenings. Importantly, the use of AI also reduced the rate of false positive results from 2.39% to 1.63%.
In addition, a higher proportion of small cancers (1 cm or less in size) were detected in the AI-assisted group (44.93%) compared to screenings without AI assistance (36.60%).
The advantage of using AI wasn’t limited to a better screening performance. Lauritzen and colleagues found that in the group screened by AI, the recall rate was decreased by 20.5% and the workload of the radiologists was reduced by 33.4%. This means that AI assistance has the potential to save cancer specialists a significant amount of time in their practice.
According to Lauritzen, more research is needed to evaluate long-term outcomes of AI-assisted cancer screenings. “Around November 2024, we will have full two-year follow-up data for the cohort of women screened with AI. In future work, we aim to quantify the effects of AI stratification, AI decision support, and radiologist access to prior screenings separately.”